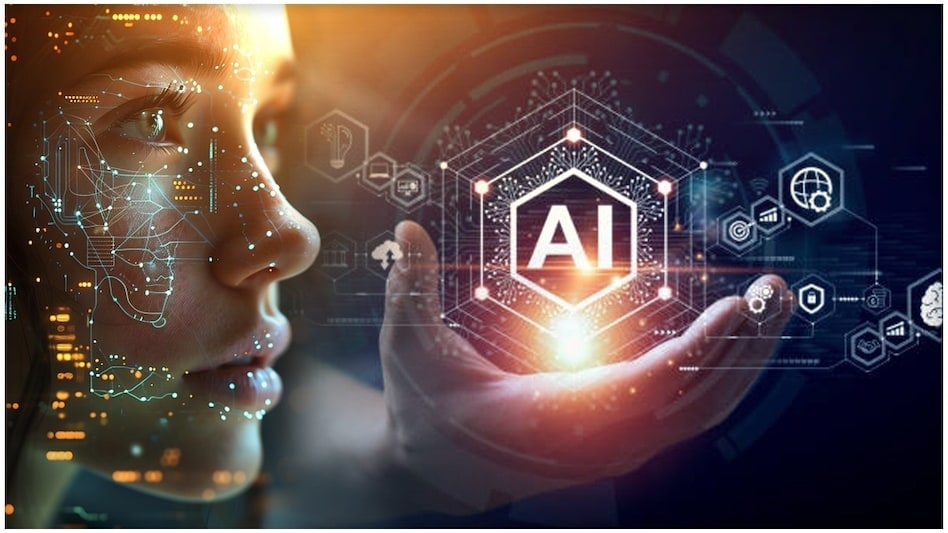
For more than a decade, artificial intelligence (AI) and machine learning (ML) have been unleashing new capabilities for enterprises and researchers. Whether it’s using predictive analytics to forecast equipment maintenance, computer vision tools to give eyes to automated assembly line robots, or digital twins to simulate the behavior of factories, cities, and even economies, the list of AI-powered applications is long and growing longer.
But none of these breakthroughs have captured the imagination of individuals and enterprises like generative AI (GenAI). Over the past two years, the world has undergone a tectonic shift due to the emergence of the large language models (LLMs) that form the foundation of GenAI applications. The aftershocks will be felt for decades to come.
I grew up in Silicon Valley, where we were always waiting for “the big one” that could change our lives overnight. While I hear that metaphor used in big tech all the time, this time, it’s appropriate.
Our ability to ask questions of GenAI chatbots using natural language and have them produce answers by drawing from nearly the entirety of recorded human information will impact every knowledge-based pursuit performed by humans. In the future, we’ll look back and see a clear demarcation: life before LLMs and life after.
What will that future look like exactly? Here’s a look ahead to the end of the decade to imagine how we’ll settle into partnership with tomorrow’s AI technologies.
1. AI models will replace enterprise operating systems and applications
Today, we use a portfolio of applications to perform basic functions such as searching databases, sending messages, or creating documents, using the tools we know well. In the future, we’ll ask an AI-based executive agent to provide an answer or perform these tasks, and it will recruit models that have proved to be safe and compliant, write or rewrite applications on the fly, and negotiate terms and conditions in your best interest. Your AI agent will simultaneously solve equations of physics, economics, law, and more to decide the best way to implement each sequence of tasks, orchestrating other models, and seeking information from additional sources as needed. The AI agent will also remember past requests and anticipate future ones, adapting to users’ behavior and creating highly personalized systems for them.
2. AI model generation and operations will become more transparent
Today, data scientists and AI engineers building leading AI models are often at a loss to explain how they reach any particular outcome. The sheer scale of inputs, the nature of training, and the massive power of computation required to produce a model all combine to make AI models inexplicable and unexplainable. While in some cases that’s perfectly acceptable, when it comes to adoption for a specific use in a highly regulated enterprise, transparency is going to be the key to adoption.
As these models become increasingly important in critical decision-making, we will see an iterative process of legislation, litigation, negotiation, and innovation among regulators, enterprises, and the communities they operate in. This process will likely continue to reflect differences in risk tolerances, values, and priorities from industry to industry and from region to region.
AI models will also need to become more transparent about the resources they consume. You can’t talk about the future of AI without considering the unprecedented amounts of electricity, water, talent, and money required to train a leading-edge model. While the eye-watering amount of resources going into training is top of mind today, we should prepare ourselves for that to continue increasing. Current leading social media infrastructure is scaled to hundreds of thousands of inferences per user-hour, but what resources will be required to support millions of inferences every hour of every day for 8 billion humans?[1]
Operators of foundational models will need to be explicit about the provenance of the energy, infrastructure, and information of their models, allowing organizations to make informed decisions about whether the insights AI models offer are worth the cost.
3. Sustainability will become a global priority — and AI will help us get there
Every element within the world’s computing infrastructure — every component in every rack in every data center — will need to be optimized for sustainability. Decision-makers will be called upon to determine whether the value of each business outcome outweighs the energy expenditure required to produce it. From mining the minerals, manufacturing the infrastructure, and deploying it at scale to bring together the information and energy to train and infer results, we’ll have to account for every joule of energy, every byte of information, and every liter of water used.
4. Building new LLMs will require new computing paradigms
Today’s most advanced LLMs are scaling to trillions of parameters, the number of variables that can be adjusted to make the model’s predictions more accurate. The open question is whether more parameters will yield even better performing models. If so, the next generation of models will require orders of magnitude more parameters, along with even larger volumes of data and gigawatts of computing power.
Research institute Epoch AI estimates that the most expensive model to date, Gemini Ultra, has a combined capital and operational cost of $800 million.[2] If the current pace of LLM development continues, within a decade, we could be spending the equivalent of the annual global IT budget to train one model at a time. In other words, we will hit a limit on our ability to train larger models using existing technologies. Even if novel technologies and algorithms begin to approach the training efficiency of biological intelligences, inferencing over these models, up to millions of times per hour for each of 8 billion people, will be an even greater hurdle. Can we afford to give everyone access to an AI-optimized future?
Photonic computation, which uses light waves for data storage and processing, could enable us to build low-latency, low-energy devices for performing inference at the edge. But training the next generation of LLMs will likely require technologies and algorithms that are still being incubated by research teams. The ultimate goal is to make AI capable of true deductive reasoning. Physics-based accelerators may be the key to a new dimension of AI behaviors that eventually lead us to artificial general intelligence.
5. AI’s biggest impact will be on human behavior
Just as humans have adapted to computers, the internet, and smartphones over the past three decades, we must adapt to AI and learn how to use it effectively. Every team and every team member should explore the possibilities of this technology and ask themselves if what they’re doing today could be done more effectively and efficiently using this technology.
The answer won’t always be yes, but every individual within every organization must be willing to seriously ponder the question. While I don’t think robots are coming for our jobs, I strongly believe that if you want to be proficient in science, engineering, industry, or even the arts, you’ll need to be proficient in AI. If you don’t know how to take advantage of this technology, you may find yourself being replaced by someone who does.
Views are personal. The author is HPE Fellow and Chief Architect at Hewlett Packard Labs
Copyright©2025 Living Media India Limited. For reprint rights: Syndications Today